Table Of Content
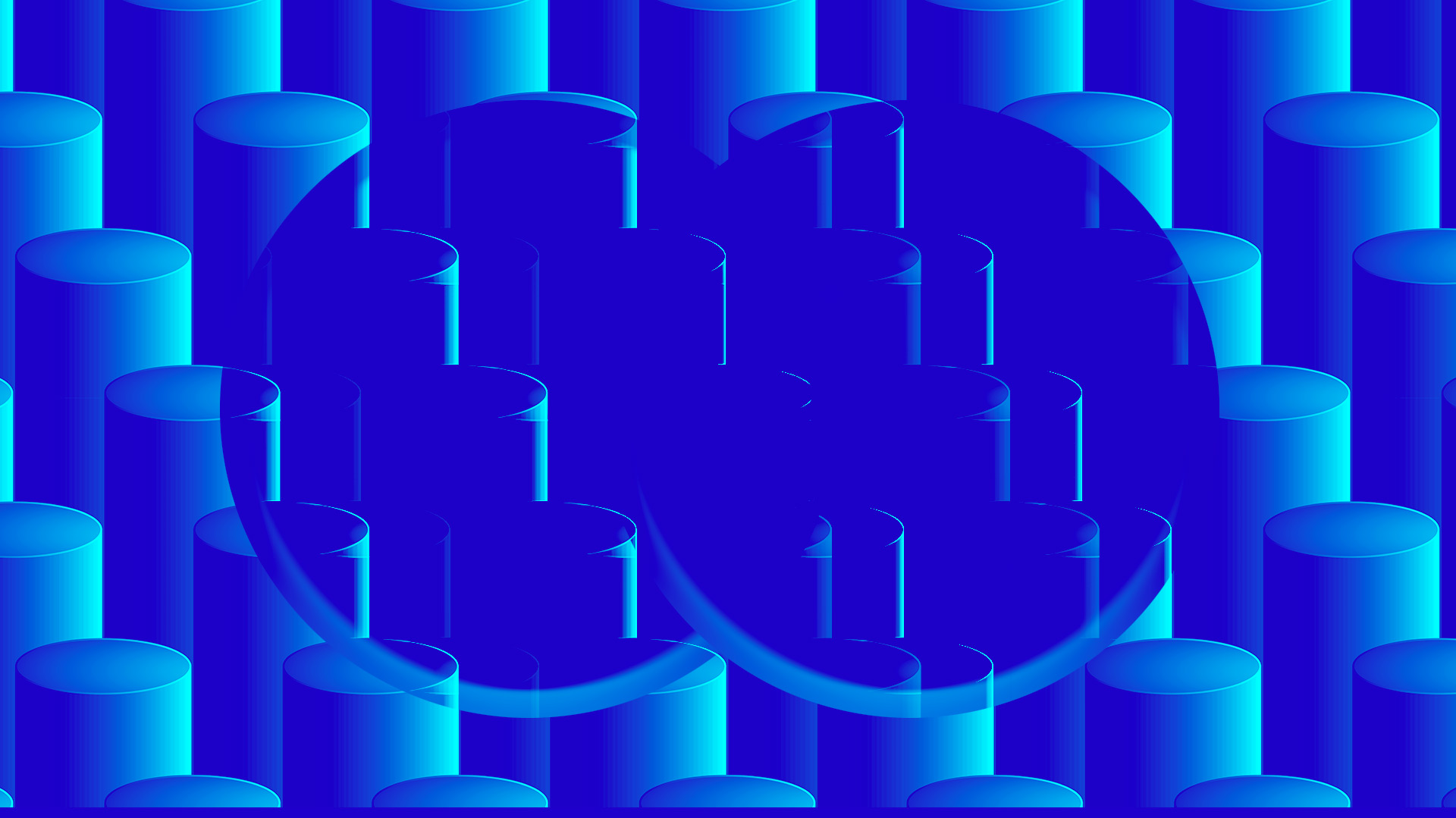
We have not randomized these, although you would want to do that, and we do show the third square different from the rest. The row effect is the order of treatment, whether A is done first or second or whether B is done first or second. So, if we have 10 subjects we could label all 10 of the subjects as we have above, or we could label the subjects 1 and 2 nested in a square. This is similar to the situation where we have replicated Latin squares - in this case five reps of 2 × 2 Latin squares, just as was shown previously in Case 2.
ANOVA and Mixed Models:
This is followed by a second treatment, followed by an equal period of time, then the second observation. The simplest case is where you only have 2 treatments and you want to give each subject both treatments. Here as with all crossover designs we have to worry about carryover effects.
Our favorite product stats, features and projects in 2022 - Rest of World
Our favorite product stats, features and projects in 2022.
Posted: Thu, 22 Dec 2022 08:00:00 GMT [source]
ANOVA: Yield versus Batch, Pressure
The varieties were applied to the main plots and the manurial treatments to the sub-plots. In determining the significance of variety the above F-value and p-value is correct. We have 40 observations (5 per variety), and after accounting for the model structure (including the extraneous blocking variable), we have \(28\) residual degrees of freedom. When we have missing data, it affects the average of the remaining treatments in a row, i.e., when complete data does not exist for each row - this affects the means.
Example 8-10: Rice Data (Experimental Design)
In this experiment, each specimen is called a “block”; thus, we have designed a more homogenous set of experimental units on which to test the tips. A block is characterized by a set of homogeneous plots or a set of similar experimental units. In agriculture a typical block is a set of contiguous plots of land under the assumption that fertility, moisture, weather, will all be similar, and thus the plots are homogeneous.
Blocking (statistics)
The incidence matrix of a non-binary design lists the number of times each element is repeated in each block. Ok, with this scenario in mind, let's consider three cases that are relevant and each case requires a different model to analyze. The cases are determined by whether or not the blocking factors are the same or different across the replicated squares.
Here is a plot of the least square means for treatment and period. We can see in the table below that the other blocking factor, cow, is also highly significant. Again, for any unbalanced data situation, we will use the GLM. For most of our examples, GLM will be a useful tool for analyzing and getting the analysis of variance summary table. Even if you are unsure whether your data are orthogonal, one way to check if you simply made a mistake in entering your data is by checking whether the sequential sums of squares agree with the adjusted sums of squares. If this point is missing we can substitute x, calculate the sum of squares residuals, and solve for x which minimizes the error and gives us a point based on all the other data and the two-way model.
The final step in the blocking process is allocating your observations into different treatment groups. All you have to do is go through your blocks one by one and randomly assign observations from each block to treatment groups in a way such that each treatment group gets a similar number of observations from each block. The next thing you need to do after you determine your blocking factors is allocate your observations into blocks.
The selection of blocking variables should be based on previous literature. Many such cases are discussed in.[7] However, it can also be observed trivially for the magic squares or magic rectangles which can be viewed as the partially balanced incomplete block designs. We have four different varieties of rice; varieties A, B, C, and D. So, imagine each of these blocks as a rice field or patty on a farm somewhere.
General balanced designs (t-designs)
Unfortunately nuisance variables often arise in experimental studies, which are variables that effect the relationship between the explanatory and response variable but are of no interest to researchers. For example you might have 5 levels of a factor, but when you split your block into plots, you can only create 3 plots. We have 3 varieties of oats, and 4 levels of fertilizer (manure). To each set of 3 plots, we’ll randomly assign the 3 varieties, and to each set of subplots, we’ll assign the fertilizers. The yield of oats from a split-plot field trial using three varieties and four levels of manurial treatment. The experiment was laid out in 6 blocks of 3 main plots, each split into 4 sub-plots.
We consider an example which is adapted from Venables and Ripley (2002), the original source isYates (1935) (we will see the full data set in Section 7.3). Atsix different locations (factor block), three plots of land were available.Three varieties of oat (factor variety with levels Golden.rain, Marvellousand Victory) were randomized to them, individually per location. Gender is a common nuisance variable to use as a blocking factor in experiments since males and females tend to respond differently to a wide variety of treatments. So far we have discussed experimental designs with fixed factors, that is, the levels of the factors are fixed and constrained to some specific values. In some cases, the levels of the factors are selected at random from a larger population. In this case, the inference made on the significance of the factor can be extended to the whole population but the factor effects are treated as contributions to variance.
In the introductory example, a blockwas given by an individual subject. Depending on the nature of the experiment, it’s also possible to use several blocking factors at once. However, in practice only one or two are typically used since more blocking factors requires larger sample sizes to derive significant results. By placing the individuals into blocks, the relationship between the new diet and weight loss became more clear since we were able to control for the nuisance variable of gender. One common way to control for the effect of nuisance variables is through blocking, which involves splitting up individuals in an experiment based on the value of some nuisance variable.
No comments:
Post a Comment